|
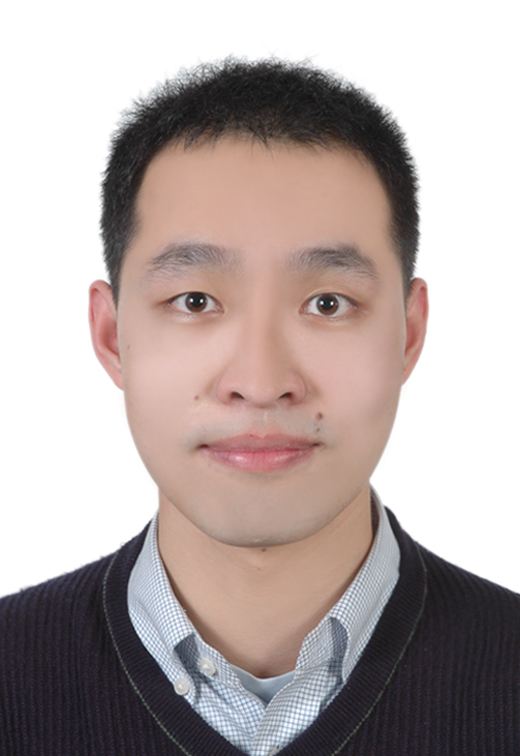
彭鑫
上海市浦江人才计划入选者 上海市青年科技英才扬帆计划入选者
彭鑫,男,1987年出生。工学博士。研究员,博士生导师。2017年度“博士后国际交流计划”派出项目获选者,2018年度上海市青年科技英才扬帆计划项目获选者。从事工业过程智能建模、控制及优化;机器学习、智能优化方法及其工业应用方面的研究,发表论文被SCI/EI收录42篇;先后承担国家自然科学基金面上项目及青年项目各1项,参与包括国家自然科学基金重大项目,国家重点研发计划等9项。
基本信息: 所属部门:自动化系 办公地址:实验19楼1404 办公电话:021-64253619 电子邮箱:xinpeng@ecust.edu.cn
主讲课程: 《最优化方法》,《人工智能基础及应用》 科研方向: 工业过程智能建模、控制及优化;全流程安全环境足迹监控与溯源诊断;机器视觉及其工业应用;机器学习、智能优化方法及其工业应用 学术兼职: IEEE会员,IES会员,中国过程系统工程学会会员
科研项目: [1] 化工园区污水系统负荷优化与运行工况评价方法研究,国家自然科学基金面上项目,62173145,主持 [2] RNA单分子检测信号的碱基特征识别方法研究,国家自然科学基金青年项目,61803157,主持 [3] 面向大规模多源异构数据的炼油过程分布式特征提取和异常识别方法研究,上海市青年科技英才扬帆计划,18YF1405200,主持 [4] 基于动态风险云图的危险化学品风险评价与预警技术,国家重点研发计划子课题,2018YFC0809304,子课题负责人 [5] 博士后科学基金会“博士后国际交流计划派出”奖学金(中方30万+德方30万),人力资源和社会保障部,中国博士后科学基金会,20170096,主持 [6] 面向炼油过程的多源数据的分布式特征提取和异常识别,中央高校基本科研业务费,222201814041,主持 [7] 城市污水处理过程异常工况预警与自愈控制,国家自然科学基金重大项目,61890933,主要参与人 [8] 化工过程建模与运行优化,国家自然科学基金杰青项目,61925305,主要参与人 [9] 基于人机合作互学习的炼油生产风险预警与智能决策,国家自然科学基金应急管理项目,61751305,主要参与人 [10] 高通量分子筛合成系统数据挖掘技术研究,中国石油化工股份有限公司,技术负责人 [11] 高通量分子筛系统的数据挖掘与应用,中国石油化工股份有限公司上海石油化工研究院,技术负责人 代表性论文: Huang H, Li Z, Peng X*, Ding S X, et al. Gaussian Process Regression with Maximizing the Composite Conditional Likelihood[J]. IEEE Transactions on Instrumentation and Measurement, 2021: 1-1. Lu Y, Peng X*, Yang D, Jiang C, et al. The probabilistic discriminative time-series model with latent variables and its application to industrial chemical process modeling[J]. Chemical Engineering Journal, 2021, 423: 130298. Huang H, Song Y, Peng X*, Ding S, et al. A Sparse Nonstationary Trigonometric Gaussian Process Regression and its Application on Nitrogen Oxides Prediction of the Diesel Engine[J]. IEEE Transactions on Industrial Informatics, 2021: 1-1. D. Tan, X. Peng*, Q. Wang, et al. Automatic Determining Optimal Parameters in Multi-Kernel Collaborative Fuzzy Clustering based on Dimension Constraint [J]. Neurocomputing, 2020, 385: 63-79. Lu Y, Peng X*, Yang D, Yang M, et al. Model-Agnostic Meta-Learning with Optimal Alternative Scaling Value and Its Application to Industrial Soft Sensing[J]. IEEE Transactions on Industrial Informatics, 2021:1-1. Peng X, Ding S X, Du W L, Zhong W M, et al. Distributed process monitoring based on canonical correlation analysis with partly-connected topology[J]. Control Engineering Practice, 2020, 101: 104500. Li L, Ding S, Peng X*. Optimal Observer-based Fault Detection and Estimation Approaches for T-S Fuzzy Systems[J]. IEEE Transactions on Fuzzy Systems, 2020: 1-1. Peng X, Li Z, Zhong W M, Qian F, et al. Concurrent Quality-Relevant Canonical Correlation Analysis for Nonlinear Continuous Process Decomposition and Monitoring[J]. Industrial & Engineering Chemistry Research, 2020, 59 (18): 8757-8768. Peng X, Tang Y, Du W L, Qian F. Multimode Process Monitoring and Fault Detection: A Sparse Modeling and Dictionary Learning Method[J]. IEEE Transactions on Industrial Electronics, 2017, 64 (6): 4866-4875. Peng X, Tang Y, Du W L,Qian F. Online Performance Monitoring and Modeling Paradigm Based on Just-in-Time Learning and Extreme Learning Machine for a Non-Gaussian Chemical Process[J]. Industrial & Engineering Chemistry Research, 2017, 56 (23): 6671-6684. Peng X, Tang Y, Du W L,Qian F. Performance monitoring of non-gaussian chemical processes with modes-switching using globality-locality preserving projection[J]. Frontiers of Chemical Science and Engineering, 2017, 11 (3): 429-439. Peng X, Tang Y, He W, Du W, et al. A Just-in-Time Learning Based Monitoring and Classification Method for Hyper/Hypocalcemia Diagnosis[J]. IEEE/ACM Trans Comput Biol Bioinform, 2018, 15 (3): 788-801. Peng X, Tian Y, Tang Y, Du W, et al. An online performance monitoring using statistics pattern based kernel independent component analysis for non-Gaussian process[C]. IECON 2017 - 43rd Annual Conference of the IEEE Industrial Electronics Society. 2017: 7210-7216. Chen X, Zhong W M, Jiang C, Li Z,Peng X*, Cheng H*. Key performance index estimation based on ensemble locally weighted partial least squares and its application on industrial nonlinear processes[J]. Chemometrics and Intelligent Laboratory Systems, 2020, 203: 104031. Hong H F, Jiang C, Peng X*, Zhong W M*. Concurrent Monitoring Strategy for Static and Dynamic Deviations Based on Selective Ensemble Learning Using Slow Feature Analysis[J]. Industrial & Engineering Chemistry Research, 2020, 59 (10): 4620-4635.
|
网页发布时间: 2016-10-20
|
|
|